The customer taking care of some critical needs and wants in a time-effective manner is the linchpin of the convenience retail channel. And what could be more frictionless than never having to interface with the checkout process—literally grab and go?
Amazon has been exploring its frictionless “Just Walk Out” technology concept since it opened its first public Amazon Go store in January 2018. Amazon has a network of about 26 Amazon Go convenience stores, ranging in size from a little over 450 square feet to 2,000 square feet. It has expanded into the grocery channel with its single Seattle Amazon Go Grocery location and plans to open grocery stores in Irvine and Woodland Hills, California, and Philadelphia this year.
Amazon Go’s technology identifies the customer upon entering the site through a smartphone app or loyalty card linked to a payment vehicle. Computer vision—a combination of cameras, shelf sensors and AI technology—takes over to “watch” the customer while shopping. The system notices if an item is picked up and if it is placed back on the shelf. And when the shopper leaves, it calculates the purchase and charges for any items carried out of the store. Amazon’s technology doesn’t identify customers’ faces. In a Los Angeles grocery store slated to open later this year, Amazon plans to use “Dash Carts” equipped with computer vision cameras that track items as customers add them to the basket. Customers sign in by scanning a QR code in the Amazon app and can skip the checkout line.
This lower-touch aspect is an appealing format in the COVID-19 pandemic environment.
These solutions offer the possibility of unattended stores, though a more common model (such as that used by Amazon Go) would have some associates at the site to facilitate customer interactions and general store operations. A live cashier is available for customers not taking part in the program. This lower-touch aspect is an appealing format in the COVID-19 pandemic environment—for however long that remains a primary concern.
Vision Tech on the Move
While Amazon was a first mover, the frictionless concept is spreading. The Japan Times has reported that the East Japan Railway Co. is expanding from its first unmanned convenience store, opened in March, to a planned 100 within the next four years. Each store uses about 50 cameras via its joint venture partner Touch To Go Co, to facilitate the shopping experience.
In the U.K., grocery giant Tesco has invested $22 million in the startup Trigo to support its development of video-driven cashierless checkout technology. And Trigo is not alone. Competitors include AiFi, Caper, GetZippin, Grabango, Mashgin, Pensa Systems and Standard Cognition—among others. Amazon itself in late March announced it is licensing its Just Walk Out technology to other retailers.
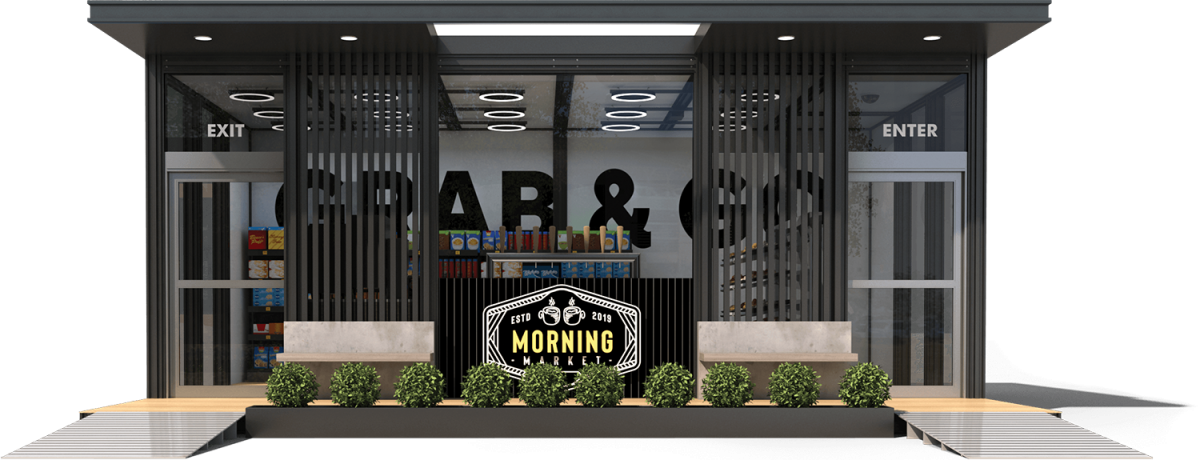
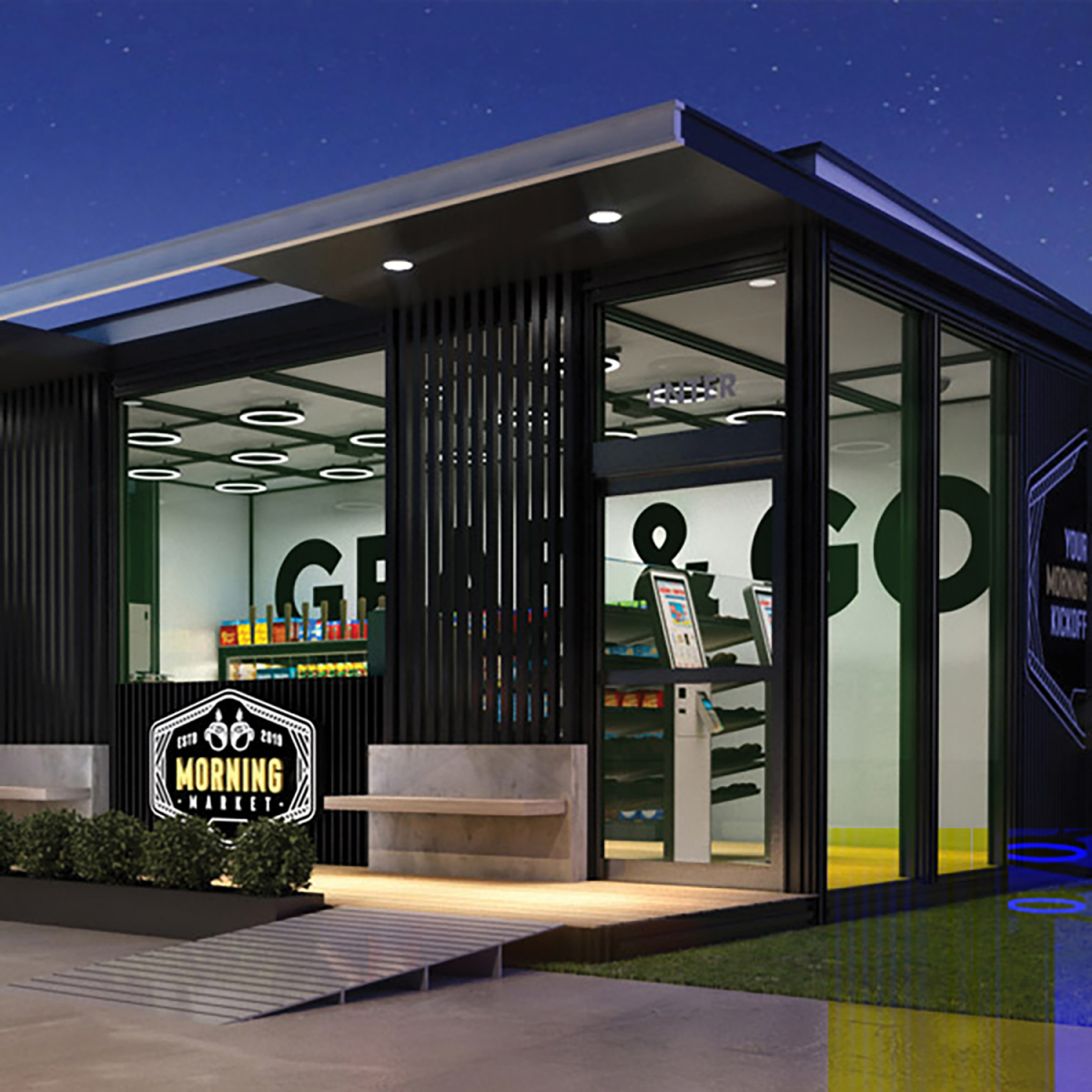
Accel Robotics is a San Diego-based startup developing computer vision software for a concept cashierless grab and go store.
Accel Robotics is one of the startups in the space with a focus on the convenience channel. The San Diego-based company late last year raised $30 million in Series A funding to finalize the development of its technology. The company is testing its frictionless commerce platform in a range of retail formats—convenience stores, grocery stores, drugstores and quick-service restaurants—though it declined to offer details due to various NDAs with its partners. It hopes to launch its solution in the first quarter of 2021.
“We have cameras that track people, we have cameras that track products, and we link that together in a very efficient way,” said Brandon Maseda, Accel Robotics co-founder and CEO. “We’re at a point now where you can almost do a full c-store with only one computer.”
Maseda noted that much of the current development is not just focused on the technology but on the shopping experience. “What’s the right shopping flow for customers? Should there be a cashier there? Those are all things that we are exploring and learning along with our customers around the world in different environments,” he said.
Accel Robotics developed a “magic box” that allows the retailer to scan in products so that the system can identify them in the store. The company determined the best approach was to leave the product onboarding up to the retailer and not develop a third party, centralized database approach. Additionally, the company is looking at opportunities to deliver convenience transactions on the forecourt.
We have cameras that track people, we have cameras that track products, and we link that together in a very efficient way.
Meanwhile, Atlanta-based NCR showcased a computer vision technology demonstrator at last year’s NACS Show. “The prototype we showed was designed to solve a specific challenge—take new ‘frictionless’ checkout technologies such as machine learning and computer vision and design a better customer experience,” said Steven O’Toole, NCR retail global head of convenience and fuel retail sales. “Our innovation team created a tabletop computer-vision-enabled checkout to fit the footprint of a typical c-store counter and used a minimum hardware investment.”
The prototype can identify multiple items at once, so shoppers can place their purchase on the counter for their basket to be identified. They can then use their mobile phone to complete the payment. “We added a tap-out station to build in an added layer of fraud reduction, preventing the sale from finalizing before a shopper taps their phone to the sensor,” he said. “The technology would also work with more traditional payment methods such as cash and card.”
Conexxus, an organization dedicated to the development and implementation of standards, technologies, innovation and advocacy for the convenience retailing marketplace, views this technology as having a three- to five-year time horizon. “Conexxus is looking to identify any standards that can be created to be ready to test and implement the technology when it becomes more mainstream,” said Gray Taylor, Conexxus executive director.
From a privacy standpoint, we have gone into this market thinking that we need to be able to perform without using facial recognition or that type of personal identifiable information.
Yet even these frictionless solutions still have some friction. In most cases, the customer must activate an app or use a card to link the transaction to the customer and payment vehicle. Facial recognition tying customers to payment when they enter the store, however, would be frictionless.
While some of the startups in computer vision checkout are exploring facial recognition capabilities, most are not, or at best they list it as a potential alternative among other approaches. Consumers who have grown up in the latter part of the digital age might not have an issue trading privacy for convenience, but plenty will have a problem, and a lot will depend upon how the facial recognition is implemented. Most of the frictionless checkout solution providers are focusing, at least initially, on smartphone app or card-based payment identifiers that offer the fewest concerns.
“There are obviously opportunities to leverage facial recognition in the future, and even more so with the COVID-19 concerns,” said Maseda. “But from a privacy standpoint, we have gone into this market thinking that we need to be able to perform this type of shopping solution without using facial recognition or that type of personal identifiable information. From our side, we are fully anonymous. We see everything as just a shopper session.”
Not So Fast
While computer vision technology is impressive, there are impediments to its adoption.
Fraud and theft are two concerns, as was the case with more traditional cashierless approaches where research shows an increase in shrink. Solution providers maintain that the sheer number of cameras and the detailed monitoring process almost eliminate the opportunity for shoplifting. In fact, solution provider Grabango states that “potential shoplifters are charged for all of the items on their person, even if hidden from view at checkout” without any confrontation.
The most pressing issue is cost. While reducing labor costs is a significant offset, the cameras, sensors, servers and other tech are cutting edge and so typically lack the economies of scale that come with mass deployments. Maintenance costs to keep the tech online are another consideration, along with additional costs to construct new stores or retrofit existing ones to support the technology.
RBC analyst Mark Mahaney outlined the cost factors relative to Amazon Go technology in a Forbes article from January 2019. He estimated that the tech cost $1 million per location and that each location would generate $1.5 million in [gross] sales. Mahaney projected a two-year ROI with the format, which provides a reasonably compelling argument in favor of the concept—if the estimates are correct—but that hinges on access to financing and how viable the concept is at locations that do not fit an upscale, urban c-store model.
It gets very easy to turn on demos and showcase this type of technology, but to deliver it at a price point that is going to make it commercially viable is a big challenge.
A new report from the User Experience Strategies Service (UXS) at Strategy Analytics noted that the Amazon Go experience provided a proof of concept, but for now it was limited beyond grocery and convenience and has some impediments in those markets.
Lisa Cooper, USX director, said the concept as it stands isn’t viable for the general convenience channel. “At this time, the costs of implementation and the requirements that would be forced on the customer (credit card/bank account setup) don’t justify the costs,” Cooper said. “You would need to have close to 100% of customers signed up, and the store must be willing to swallow gargantuan upfront costs for setup, plus significant ongoing maintenance costs with all the processing required to run the system in real time.”
AiFi claims its solution can scale through the range of retail, and Accel Robotics has put cost reduction front and center. “I’ve seen the different companies take different approaches, and we’re focused on low cost,” Maseda said. “It gets very easy to turn on demos and showcase this type of technology, but to deliver it at a price point that is going to make it commercially viable is a big challenge. We’ve really been focusing on how we can deliver this to where it’s easy to deploy, it’s low cost, and it makes economic sense for all sides.”
Most of the solution providers, Accel Robotics included, are exploring the range of models to provide the solution, including software-as-a-service models. It also is logical that costs will drop as the tech matures and economies of scale begin to develop.
Computer-vision-aided technology also is competing with less exotic solutions that offer slightly more friction. Kroger, Sam’s Club and 7-Eleven have introduced scan-and-go services where the customer scans the barcodes using a smartphone and an app tied to payment.
“I've tried those shopping experiences myself, and it felt for me like you’re putting the labor on the customer,” said Maseda. “You have to balance that, obviously, with the cost of deploying a system like ours. But that’s why we’ve focused on reducing the cost. Hey, how can we deliver this where we keep the costs low to set up and then make shopping really easy for the consumers?”
The Many Facets of Facial Recognition
One-to-many is a facial recognition approach common with government/police applications. Cameras scan a crowd and identify specific people, making it one of the most controversial applications of the technology, with companies like Amazon, IBM and Microsoft recently stepping back from their support and development.
However, one-to-many has been making inroads into retail in specific applications. For example, FaceFirst uses the technology for security applications in large-format retail, casinos, airports, events and financial institutions. It maintains a database to identify known shoplifters, criminals, persons of interest and other threats. Facewatch, a similar solution in the United Kingdom, provides these capabilities to smaller retail formats and pubs.
One-to-many also can be used to provide targeted marketing to specific customers while roaming the aisles, and it can facilitate frictionless checkout. But it does raise privacy concerns, since it requires the collection and storage of customer identifiers.
A one-to-one approach involves a specific person being identified by a specific device, such as a smartphone (to unlock access) or a shopping terminal with a camera to facilitate checkout. This can be private in the case of a smartphone, where the identifying data are exclusively kept encrypted on the device, or far less private, where the collected biometric data are centrally stored and managed in the cloud.
With Alibaba, whose “Smile to Pay” technology is already being deployed at KFC restaurants in China, customers who use the facial recognition option in the Alipay app can order and checkout at a kiosk—no smartphone required.
Facial recognition also is being used in an entirely anonymous manner. For age verification on restricted purchases, NCR’s “FastLane” self-checkout stations in the U.K. use a checkout camera to identify the general demographics of a customer and determine an age range. Customers identified as potentially under age 25 require a manual age verification, though the rest are free to grab their purchases and leave.
Walgreens also trialed anonymous facial recognition technology to identity the general demographics of customers at the cooler to develop targeted promotional offers. A growing number of states and localities regulate the collection of facial or other biometric information in retail environments. The Illinois Biometric Information Privacy Act requires companies to get approval before collecting biometric information. And a class action lawsuit was filed in September 2019 against Home Depot over its use of facial recognition for loss prevention.
There’s also evidence that facial recognition systems can misidentify people of color, women, older persons and children. A 2019 study by the National Institute of Standards and Technology (NIST) raised questions about racial bias. NIST found that algorithms for leading facial recognition systems were more likely to misidentify people of color, misidentify women more than men and elderly people and children more than other age groups.